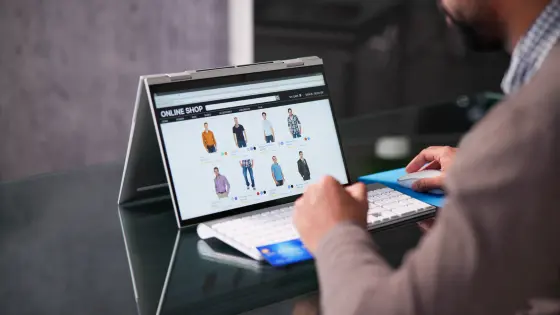
eCommerce search platforms have evolved substantially over the last 30 years. In the 1990s, they were simple tools that only displayed results based on keyword matching, and if the user made a typographical error, the tool would return no results.
As of today, 2024, these search engines are capable of understanding when a user makes a typographical error, recommending search terms, recognizing synonyms, and even analyzing user behavior to display products according to their interests.
Major platforms like Amazon, Mercado Libre, and Netflix have raised the bar for what a good search experience should be like. Today, users expect search to be just as simple, intuitive, and fast as it is on these platforms. This puts pressure on companies that want to optimize the search engine for their eCommerce site.
Search has become a relevant topic in online stores, both to improve user experience and to increase sales, which has led more and more companies to establish their own departments exclusively dedicated to improving search results.
Below, we will present the evolution of search in eCommerce and the trends your company needs to adapt to.
What Is an eCommerce Search Engine?
A search engine is a functionality integrated into an eCommerce platform that allows users to find products within an online store's catalog.
Its main objective is to facilitate product search and navigation, improving the user experience and increasing the likelihood that visitors will find and purchase the items they desire.
Search engines index the product catalog to display results based on the keywords entered in the search bar.
These search engines are an essential tool that not only helps users find what they are looking for quickly and efficiently but also plays a crucial role in the commercial success of the online store by enhancing the user experience and increasing sales.
Background of Search Engines in eCommerce
eCommerce search engines have a long history that has developed in parallel with the evolution of information technology and online commerce. Here is an overview of the background of search engines in eCommerce:
1. Origin and Keyword Search
Static Catalogs: Early eCommerce sites featured static catalogs where users navigated through categories to find products. There were no sophisticated search tools.
Basic Search (1990s): The first versions of eCommerce search engines allowed users to search for products by exact keyword matches in product titles or descriptions.
Limitations: These systems were basic and had little ability to handle typographical errors, synonyms, or variations in keywords. Results were often irrelevant if exact terms weren't used.
2. Development of Search Algorithms
Advances in Algorithms (early 2000s): Search algorithms began to evolve to improve the relevance of results. Techniques such as phrase matching, proximity analysis to evaluate the distance and relationship between words to improve result accuracy, and term weighting to identify the most relevant terms for a specific query were introduced.
Semantic Search (late 2000s): Semantic search algorithms were developed to understand the context and intent behind user queries. An example of this type of search is when entering "headache," the results would directly show a remedy for this pain. This allowed for greater accuracy in search results by interpreting the meaning of words rather than just looking for exact matches.
Spell Correction and Suggestions: Features like automatic correction of typographical errors and search suggestions were added to help users find what they were looking for even if they made mistakes while typing.
3. Integration with eCommerce Platforms
Early Integration (mid-2000s): eCommerce platforms began to integrate more advanced search engines directly into their systems. This allowed online stores to offer more sophisticated and personalized search experiences.
Filters and Facets: Filters and facets were introduced, allowing users to refine their searches by various criteria such as price, category, brand, among others. This significantly improved the search experience by enabling users to find specific products more quickly and efficiently.
Visual Search (late 2010s): Image recognition technology allowed users to search for products using photos. This innovation was driven by advances in machine learning and artificial intelligence.
Voice Search: With the popularization of virtual assistants (like Siri, Alexa, and Google Assistant), voice search capabilities were introduced on eCommerce platforms, making it easier to search on mobile devices and smart homes.
4. Current and Future Trends
Predictive Recommendations (2020s): Search engines began using Artificial Intelligence (AI) and machine learning to analyze large amounts of data and offer predictive recommendations based on user behavior patterns.
Multimodal Search: The integration of multiple search modes (text, voice, visual) into a unified and seamless experience.
Omnichannel Experience: Search engines are evolving to offer a consistent and continuous search experience across multiple channels and devices. Thanks to this, you can check inventory availability in each store or filter to show only what is available in online inventory.
Conversational Search: Chatbots and virtual assistants are being used to interact with users in a conversational manner to help them find products more naturally and efficiently.
Augmented Reality and Virtual Reality: The integration of these technologies is being used to offer more immersive search and shopping experiences. In furniture stores or clothing shops, a virtual fitting room is being integrated, allowing users to try the product from home.
The evolution of eCommerce search engines has gone through several stages, from simple keyword matching engines to sophisticated semantic and personalized search systems. Integration with eCommerce platforms has been crucial to improving the relevance and accuracy of search results, providing users with more efficient and satisfying shopping experiences.
Emerging Technologies in eCommerce Search Engines
Emerging technologies are innovative technologies that bring improvements compared to already established traditional technologies. In this case, we will refer to the emerging technologies being applied to the internal search engines of eCommerce platforms.
Artificial Intelligence
Used to improve the relevance and accuracy of search results.
Applications:
Personalization: AI is used to analyze large volumes of user data (purchase history, browsing, preferences) and personalize search results for each user.
Predictive Recommendations: AI can predict which products might interest the user based on their past behavior and offer relevant recommendations.
Sentiment Analysis: AI systems can analyze user reviews and comments to adjust the relevance of results based on the perception of the product.
Machine Learning
Used to develop models that enhance the relevance and accuracy of results.
Applications:
Search Algorithm Optimization: Search algorithms can be continuously optimized through machine learning, analyzing user behavior and adjusting results accordingly.
Product Classification and Clustering: Utilizes supervised and unsupervised learning techniques to classify and group products more effectively, making it easier for users to find similar products.
Natural Language Processing (NLP)
Used to understand, interpret, and respond to search queries in a more human and contextual manner.
Applications:
Semantic Search: Allows search engines to understand the intent behind user queries and provide more relevant results based on context and meaning, rather than just keyword matches.
Voice Search: Crucial for voice search functionality, allowing users to make spoken queries naturally and receive accurate responses.
Review and Comment Analysis: Used to analyze and extract useful information from user reviews and comments, helping to improve search results and recommendations.
Emerging technologies such as artificial intelligence, machine learning, and natural language processing are revolutionizing eCommerce search engines, making them smarter, more personalized, and efficient, significantly improving the online shopping experience.
Personalization and Recommendations in eCommerce Search Engines
Personalization and recommendations in eCommerce search engines are crucial aspects to improve user experience, increase customer satisfaction, and maximize conversion rates. This can be achieved through the following technologies:
1. Collaborative Filtering: A technique used to make recommendations based on the behaviors and preferences of multiple users. It is based on the idea that if two users have shown similar preferences in the past, they are likely to continue having similar tastes in the future.
Types of Collaborative Filtering:
User-based: Recommends products to a user based on the preferences of other users with similar tastes.
Item-based: Recommends products that are similar to those the user has interacted with before, based on the similarity between items in the preferences of all users.
2. Behavior-based Recommendations: This method uses user behavior data, such as browsing history, previous purchases, and interactions with products (clicks, time spent) to personalize recommendations.
Techniques Used:
Session Analysis: Analyzes user actions during a browsing session to make immediate and contextual recommendations.
Predictive Modeling: Uses machine learning algorithms to predict the products the user is likely to be interested in purchasing, based on their past behavior.
Real-time Recommendations: Adjusts product recommendations in real time as the user interacts with the site.
3. Personalized Search Experience: The personalized search experience adapts search results and the user interface according to individual preferences, behavior, and user profile.
Key Elements:
Personalized Results: Adapting search results to show products that are more relevant to the individual user.
Adaptive User Interface: Changes in the interface and layout of results based on user preferences and behavior.
Smart Search Suggestions: Offering search term and category suggestions based on the user's history and preferences.
Personalization and recommendations in eCommerce search engines use advanced technologies to offer highly relevant and efficient search and product discovery experiences. These techniques not only improve customer satisfaction but also drive sales and retention.
In summary, the evolution of eCommerce search engines has been remarkable, evolving from simple text search tools in the 90s to advanced systems employing artificial intelligence and machine learning today.
The way information and products are searched for online has changed, as consumers have altered their search habits, now searching within internal search engines of marketplaces, eCommerce sites, or social media. This change occurred due to the convenience and trust that users find in these different sites, thanks to the variety of product types they can find in one place.
The evolution of eCommerce search engines reflects the advancement of technology and the growing sophistication of consumer expectations. Today, your eCommerce search engine must focus on understanding user intent and providing highly relevant and personalized results, which is crucial for improving customer experience and increasing conversion rates in your online store.